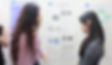
Current Research
At the Visual Cognition Lab we study the time course of visual perception. Read below for more information about the questions we ask, the methods we use and the contributions we have made.
The Questions We Ask
-
What are all the steps between opening your eyes and fully understanding the scene in front of you at the semantic level?
-
What are the information limits of visual perception?
-
What features are diagnostic of different scenes?
-
In what ways do language and visual perception interact?
The Methods We Use
We use electroencephalography (EEG) and behavioral experiments to explore how the brain processes visual information. By recording brain activity with EEG, we can track neural signals in real time and analyze how they relate to perception. We also use decoding and mutual information techniques to uncover patterns in these signals to understand how brain information translates to behavior. Our approach combines signal processing, machine learning, and statistical modeling to get a clearer picture of how the brain makes sense of the world.

The Contributions We've Made
Our research investigates how humans rapidly and effortlessly make sense of the world around them—within a fraction of a second. We have shown that people can grasp the meaning of a scene almost instantly, but how does this process actually work?
Dr. Greene and her lab have found that scene perception goes beyond simply recognizing objects. Instead, we rely on scene affordances—the actions a space allows—to interpret our surroundings. For example, what makes a kitchen a kitchen? It’s not just the presence of a stove or a fridge but the fact that you can cook and eat there. This ability to perceive spaces in terms of what we can do in them, rather than just identifying their contents, plays an important role in how we navigate a scene.
Additionally, global scene properties—such as layout—help us quickly understand a scene as a whole. Our research on top-down scene understanding has demonstrated that observers are significantly better at detecting typical scene configurations compared to images containing unusual/illogical characteristics.